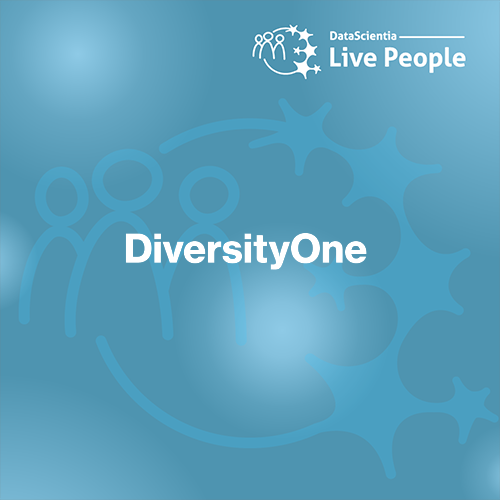
Description
DiversityOne is one of the largest and most geographically diverse datasets for studying everyday life behavior of college students through smartphone sensors and self-reports.
Key statistics
Questionnaires from 18K+ participants
Intensive longitudinal survey of 4 weeks
Data from both the Global North and the Global South
Passive smartphone sensor data and self-reports from 782 participants across eight universities in eight different countries
Raw sensor data from 26 modalities
Possible research directions, but not limited to:
- developing machine learning models across multiple domains, such as behavior recognition
- multimodal time-series modeling
- model generalization and domain adaptation across countries
- social practices understanding
- explore the diversity of daily activities in various cultural contexts
- cross-cultural studies
Dataset Description
The DiversityOne dataset was collected through a collaborative effort involving universities across eight different countries, encompassing both the Global North and Global South. This extensive data collection includes intensive longitudinal surveys and passive smartphone sensor data from diverse cultural contexts, providing a unique resource for cross-cultural studies and behavioral research.
Countries and Universities
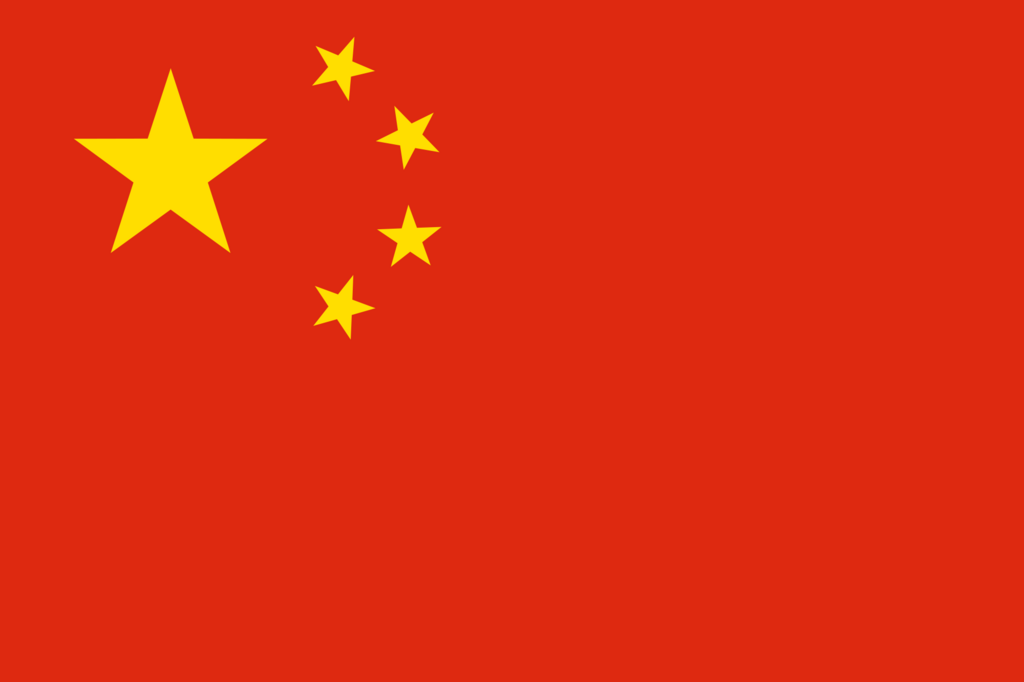
China
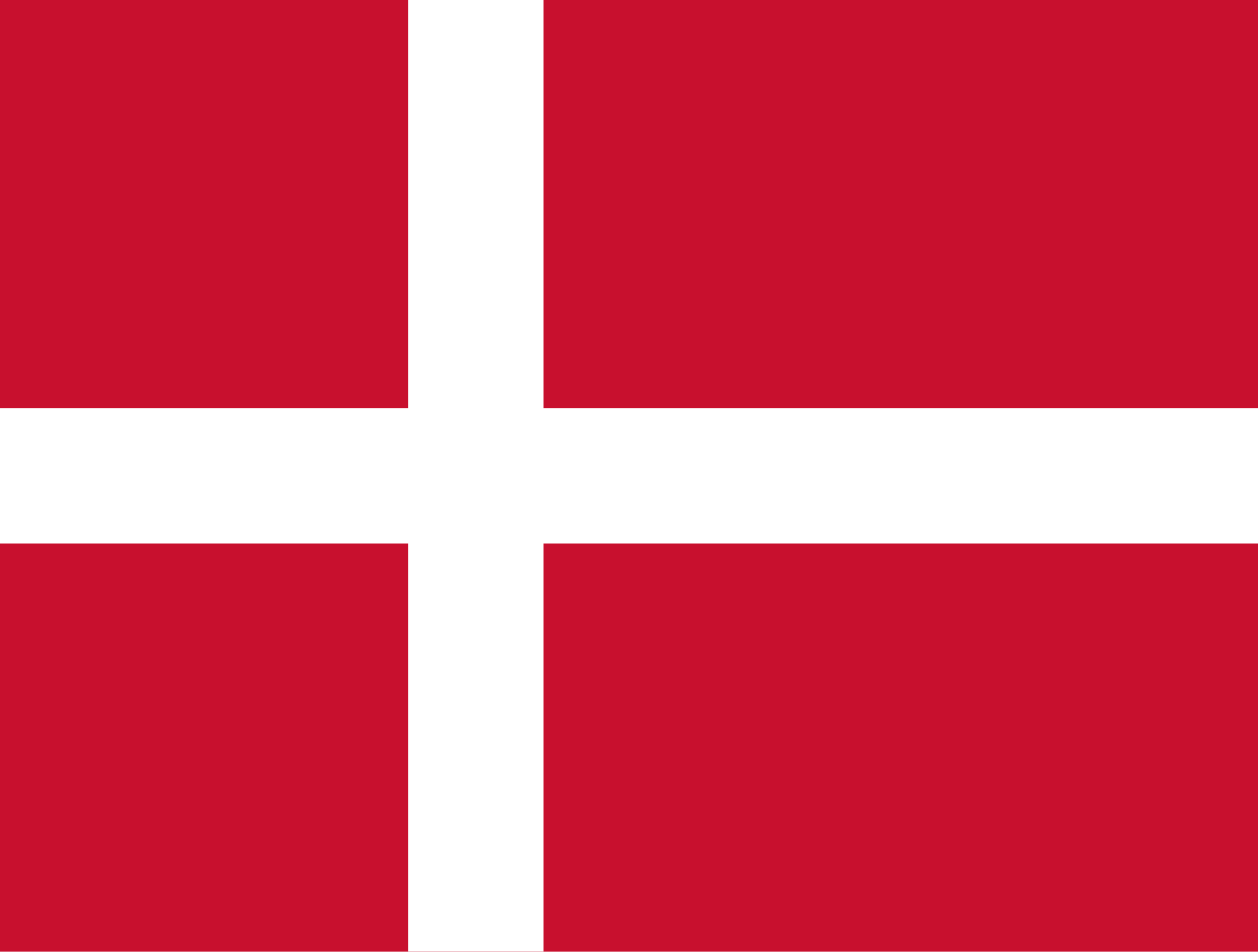
Denmark
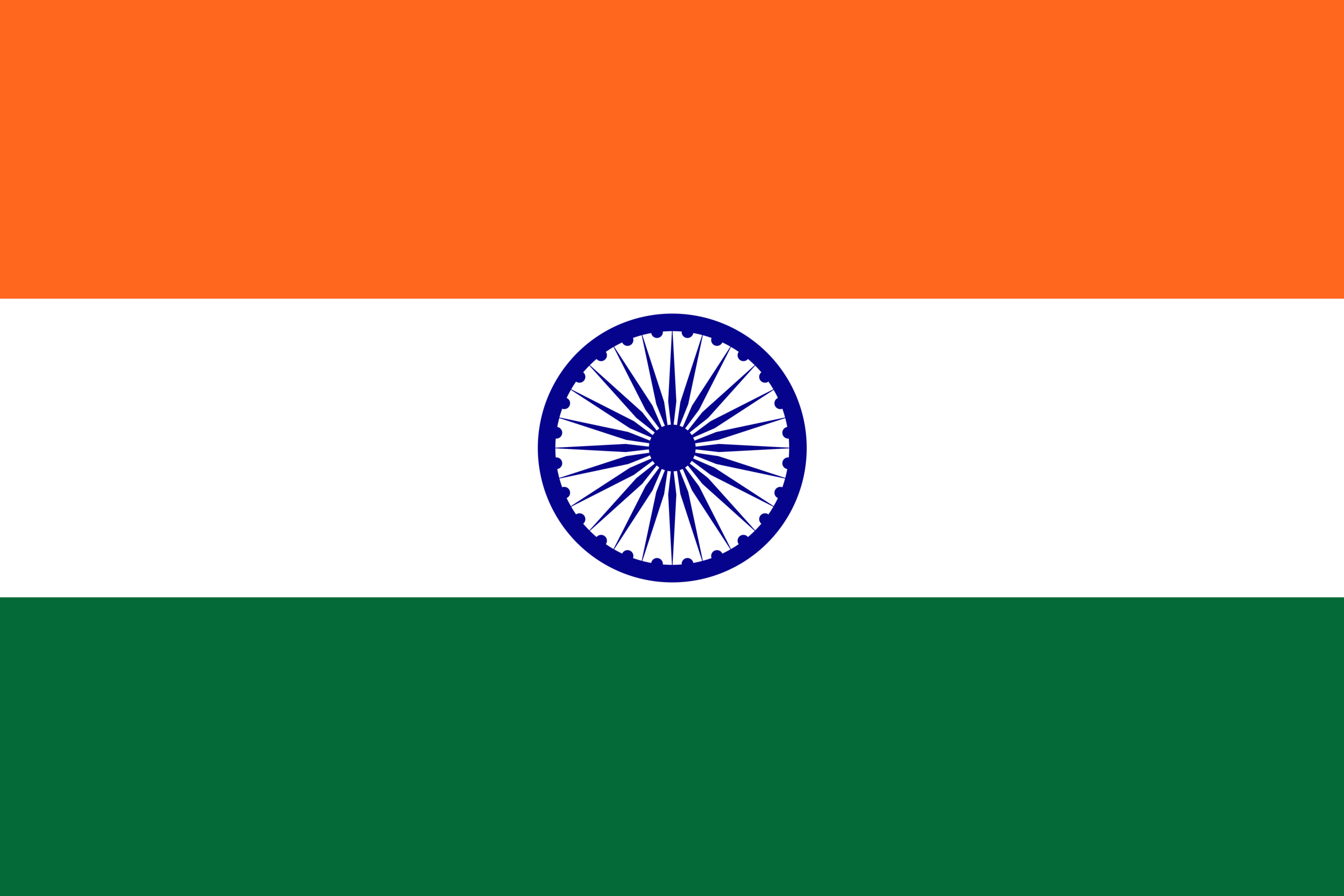
India
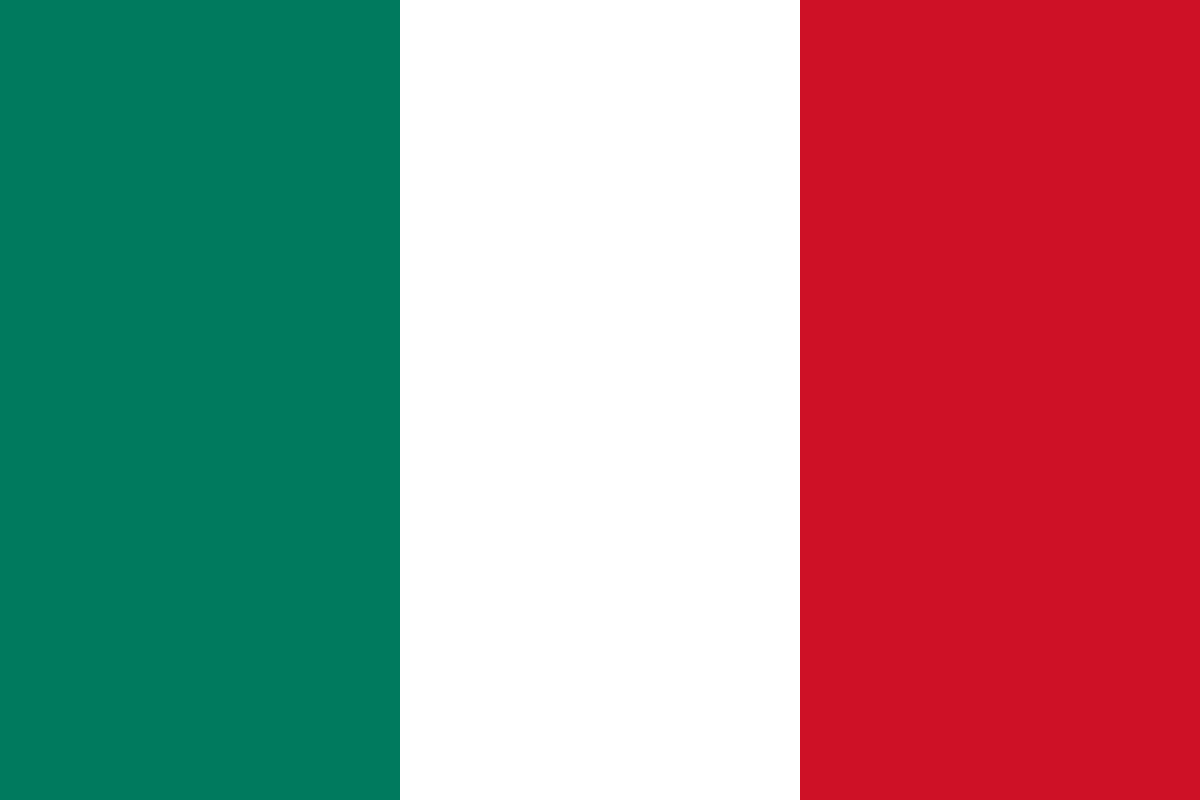
Italy
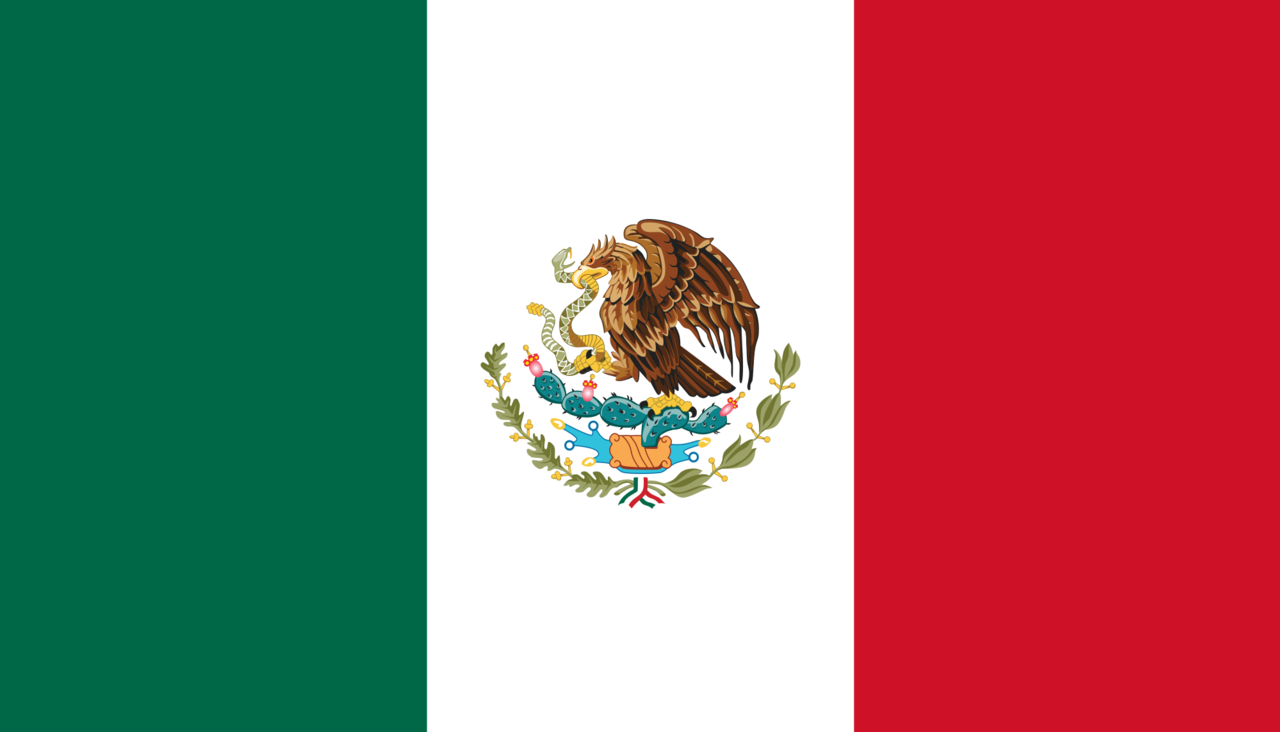
Mexico
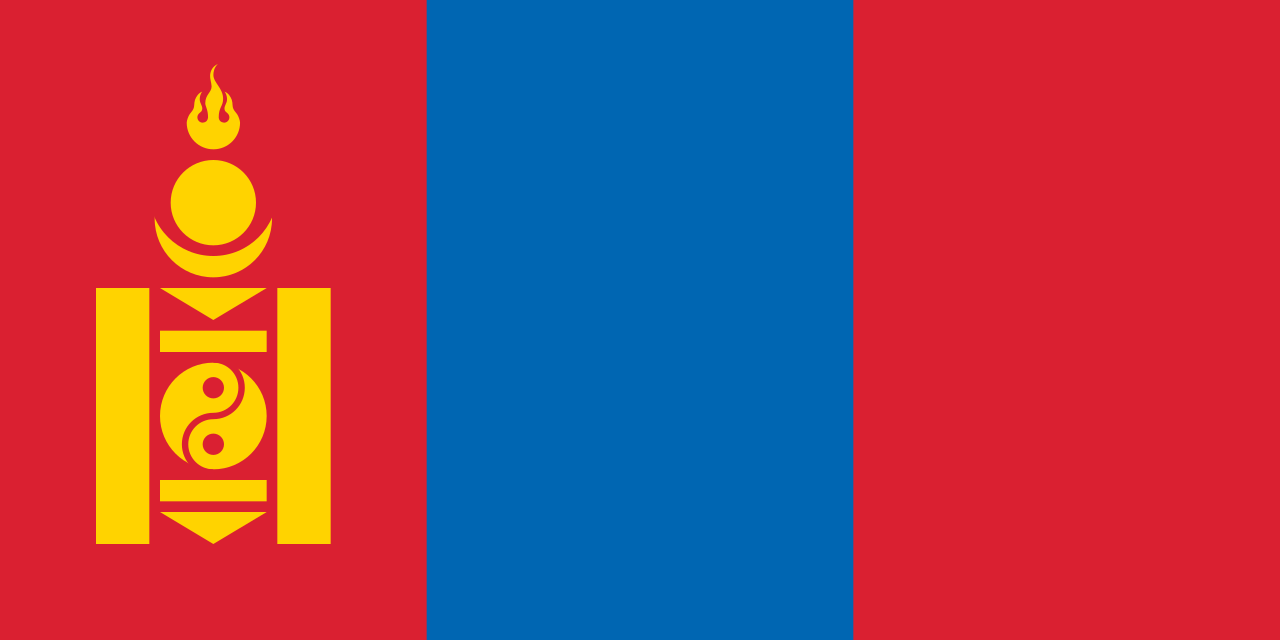
Mongolia
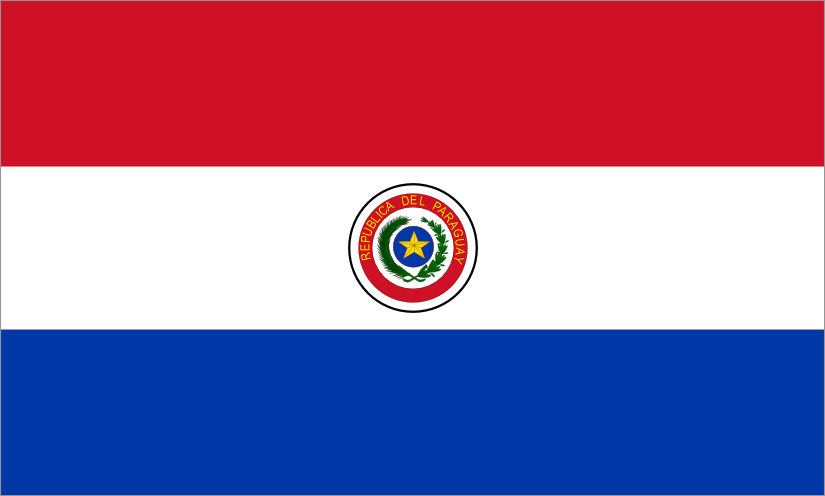
Paraguay
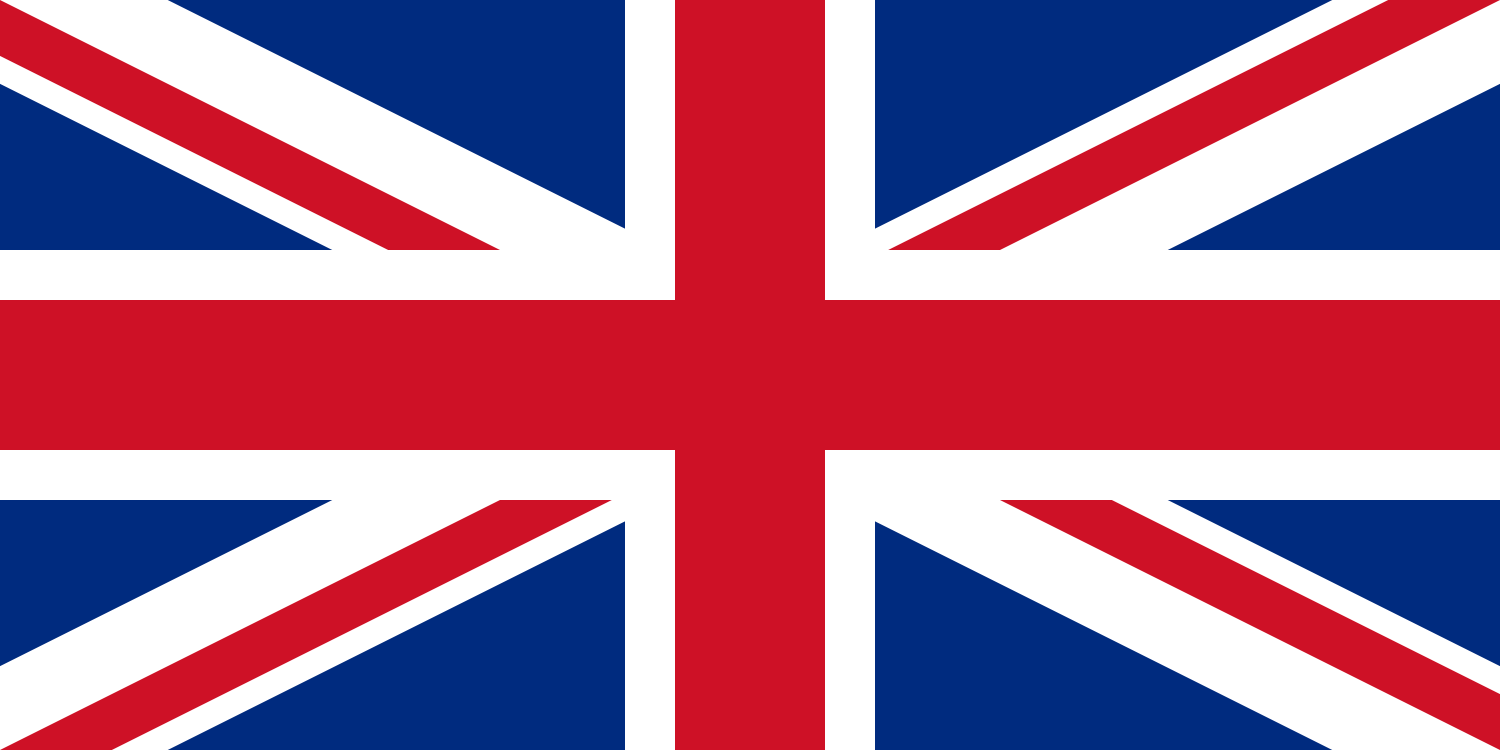
United Kingdom
The overall data collection protocol is presented in the following figure:
Figure 1: Data collection protocol
- 1st Questionnaire: it was sent to all students of the participating universities. It implements standard scales for measuring personality traits (Big Five Inventory) and attitudes toward values (Basic Values Survey). See the list on the data catalog website.
- 2nd Questionnaire: participants joining the smartphone data collection answered questions about social practices and from Jungian Scale for Personality Types and Human Values Survey scales.
- 1st Data collection phase: the intensive longitudinal survey observes human behaviors over time. Participants installed the ILog app on their smartphone. The app collects self-reports (every 30 minutes and additional morning and evening questions) and passive sensor data.
- 2nd Data collection phase: as in the 1st phase, but self-reports are asked every hour.
- 3rd Questionnaire: it collects feedback about the data collection and the Multiple Intelligence Scale.
The collected sensors are repoted in the following table.
Category | No | Type | Name | Frequency |
---|---|---|---|---|
Connectivity | 1 | SW | Bluetooth Devices | Once every minute |
2 | SW | Cellular network info | Once every minute | |
3 | SW | WIFI Network Connected to | On change | |
4 | SW | WIFI Networks Available | Once every minute | |
Environment | 5 | HW | Light | Up to 10 samples per second |
6 | HW | Pressure | Up to 10 samples per second | |
Motion | 7 | HW | Accelerometer | Up to 10 samples per second |
8 | HW | Gyroscope | Up to 10 samples per second | |
9 | SW | Movement Activity | Once every 30 seconds | |
10 | SW | Step Counter | Up to 10 samples per second | |
11 | SW | Step Detection | On change | |
Position | 12 | HW | Location | Once every minute |
13 | HW | Magnetic Field | Up to 10 samples per second | |
14 | SW | Proximity | Up to 10 samples per second | |
App usage | 15 | SW | Headset Status [ON/OFF] | On change |
16 | SW | Music Playback | On change | |
17 | SW | Notifications | On change | |
18 | SW | Running Applications | Once every 5 seconds | |
Device usage | 19 | SW | Airplane Mode [ON/OFF] | On change |
20 | SW | Battery Charge [ON/OFF] | On change | |
21 | SW | Battery Level | On change | |
22 | SW | Doze Mode [ON/OFF] | On change | |
23 | SW | Ring mode [Silent/Normal] | On change | |
24 | SW | Touch event | On change | |
25 | SW | Screen Status [ON/OFF] | On change | |
26 | SW | User Presence | On change |
Country | Acronym | 1st QU | 2nd QU | 3rd QU | iLog signed | iLog data | μ Age (σ) | % women |
---|---|---|---|---|---|---|---|---|
China | JLU | 1,007 | 69 | 41 | 54 | 44 | 19.4 (2.2) | 61 |
Denmark | AAU | 412 | 16 | 15 | 27 | 20 | 28.2 (6.3) | 58 |
India | AMRITA | 4,183 | 141 | 38 | 64 | 45 | 21.4 (2.9) | 29 |
Italy | UNITN | 5,692 | 287 | 215 | 263 | 241 | 22.1 (3.2) | 56 |
Mexico | IPICYT | 40 | 9 | 11 | 21 | 21 | 25.2 (4.1) | 33 |
Mongolia | NUM | 3,972 | 214 | 152 | 231 | 201 | 20.0 (3.1) | 65 |
Paraguay | UC | 1,342 | 33 | 25 | 43 | 28 | 23.3 (5.1) | 61 |
UK | LSE | 1,980 | 143 | 45 | 88 | 66 | 24.6 (5.0) | 66 |
Total | 18,628 | 912 | 542 | 782 | 666 |
How to get the Dataset
1. Explore the Data Catalog – Browse the data catalog to identify the datasets relevant to your research.
2. Submit a Request – Visit the DataScientia Marketplace to submit your research proposal and request access to datasets. Fill out the request form, specifying your research purpose and selecting the desired datasets.
3. Receive Approval & Download – Once your request is approved, you will receive an email with instructions on how to download the dataset.
Additional information can be found on the catalog. For any issue or question, please feel free to contact us at datadistribution.knowdive@unitn.it.
How to cite
@article{
title = {{{DiversityOne}}: {{A Multi-Country Smartphone Sensor Dataset}} for {{Everyday Life Behavior Modeling}}},
author = {Busso, Matteo and Bontempelli, Andrea and Javier Malcotti, Leonardo and Meegahapola, Lakmal and Kun, Peter and Diwakar, Shyam and Nutakki, Chaitanya and Rodas Britez, Marcelo and Xu, Hao and Song, Donglei and Ruiz-Correa, Salvador and Mendoza-Lara, Andrea-Rebeca and Gaskell, George and Stares, Sally and Bidoglia, Miriam and Ganbold, Amarsanaa and Chagnaa, Altangerel and Cernuzzi, Luca and Hume, Alethia and Chenu-Abente, Ronald and Alia Asiku, Roy and Kayongo, Ivan and Gatica-Perez, Daniel and De Götzen, Amalia and Bison, Ivano and Giunchiglia, Fausto},
journal={Proceedings of the ACM on interactive, mobile, wearable and ubiquitous technologies},
year={2025},
publisher={ACM New York, NY, USA}
}
Matteo Busso, Andrea Bontempelli, Leonardo Javier Malcotti, Lakmal Meegahapola, Peter Kun, Shyam Diwakar, Chaitanya Nutakki, Marcelo Rodas Britez,Hao Xu, Donglei Song, Salvador Ruiz-Correa, Andrea-Rebeca Mendoza-Lara, George Gaskell, Sally Stares, Miriam Bidoglia, Amarsanaa Ganbold, Altangerel Chagnaa, Luca Cernuzzi, Alethia Hume, Ronald Chenu-Abente, Roy Alia Asiku, Ivan Kayongo, Daniel Gatica-Perez, Amalia De Götzen, Ivano Bison, and Fausto Giunchiglia. (2025). DiversityOne: A Multi-Country Smartphone Sensor Dataset for Everyday Life Behavior Modeling. Proceedings of the ACM on interactive, mobile, wearable and ubiquitous technologies.
Studies based on DiversityOne
2024
- Meegahapola et al. 2024. M3BAT: Unsupervised Domain Adaptation for Multimodal Mobile Sensing with Multi-Branch Adversarial Training. Proceedings of the ACM on Interactive, Mobile, Wearable and Ubiquitous Technologies. Paper accessible here.
Keywords: social context, mood - Mäder et al. 2024. Learning About Social Context from Smartphone Data: Generalization Across Countries and Daily Life Moments. In Proceedings of the CHI Conference on Human Factors in Computing Systems. Paper accessible here.
Keywords: social context
2023
- Meegahapola et al. 2023. Generalization and personalization of mobile sensing-based mood inference models: an analysis of college students in eight countries. Proceedings of the ACM on interactive, mobile, wearable and ubiquitous technologies. Paper accessible here.
Keywords: mood - Assi et al. 2023. Complex daily activities, country-level diversity, and smartphone sensing: A study in Denmark, Italy, Mongolia, Paraguay, and UK. In Proceedings of the 2023 CHI conference on human factors in computing systems. Paper accessible here.
Keywords: daily activities - Kammoun et al. 2023. Understanding the Social Context of Eating with Multimodal Smartphone Sensing: The Role of Country Diversity. In Proceedings of the 25th International Conference on Multimodal Interaction. Paper accessible here.
Keywords: social context - Girardini et al. 2023. Adaptation of student behavioural routines during Covid-19: a multimodal approach. EPJ Data Science. Paper accessible here.
Keywords: daily routines
2022
- Bouton-Bessac et al. 2022. Your Day in Your Pocket: Complex Activity Recognition from Smartphone Accelerometers. In International Conference on Pervasive Computing Technologies for Healthcare. Paper accessible here.
Keywords: daily activities
Related links
- The dataset is the result of the European project ‘Wenet – The internet of US’. See the project on Cordis, Project website
- Datascientia projects: China, Denmark, India, Italy, Mexico, Mongolia, Paraguay, UK
News
- 2025-02-05: We are organizing an open challenge on the DiversityOne dataset at UbiComp 2025. Visit the workshop webpage for more details.
- 2025-02-03: The dataset paper has been accepted on UbiComp/Imwut.
Contacts
Contact us for any questions or issues related to the datasets.
Acknowledgments
This research has received funding from the European Union’s Horizon 2020 FET Proactive project “WeNet – The Internet of us”, grant agreement No. 823783. We deeply thank all the volunteers across the world for their participation in the study. We thank the anonymous reviewers for their valuable feedback. We acknowledge the use of ChatGPT and Grammarly as tools for grammar refinement. University of Trento, Jilin University, National University of Mongolia and Universidad Católica “Nuestra Señora de la Asunción” have participated to the work described in this paper as part of the DataScientia initiative.